Mitigating the growing threat to biometric security posed by fake digital imagery and injection attacks
Deepfakes are rendered digital imagery–like special effects in a movie–that allow a fraudster to create realistic videos and use them to spoof biometric security. Concerns about fraud are growing as the technology to create deepfakes becomes increasingly accessible.
The threat of deepfakes is substantial. New ID R&D survey data shows 91 percent of businesses acknowledge that they or their customers are concerned about deepfake fraud, with 42 percent having encountered deepfake attacks. Also, 84 percent of the same respondents express similar concern about injection attacks, with 37 percent having first hand exposure. In 2023, 20% of successful account takeover attacks will leverage deepfakes (Gartner).
This article introduces various types of deepfakes, how they are used in attacks to defeat biometric security, and how the threat is mitigated. For an even deeper dive, refer to our white paper.
Generating fraudulent imagery: deepfakes and cheapfakes
Deepfakes are a rapidly evolving type of synthetic media in which artificial intelligence (AI) is used to generate realistic-looking digital imagery that depicts existing people doing or saying things they never actually did or said, or even fabricating people that don’t actually exist. According to Idiap Research Institute, 95% of facial recognition systems are unable to detect deepfakes.
Unlike photos and screen replays that are “presented” to the camera on a physical medium (a presentation attack), deepfakes are natively digital, created using deep neural network-based machine learning. Realistic deepfakes can be “injected” into a device by way of hardware and software hacks in a way that bypasses the camera (an injection attack), which makes them difficult for presentation attack detection.
Cheapfakes are a class of fake media that is easy and quick to produce, especially for amateur users. Compared to traditional deepfakes, cheapfakes do not require sophisticated coding, manual neural network tuning, or post-production skills. Experts highlight that cheapfakes can be dangerous because they take little effort and cost to fabricate.
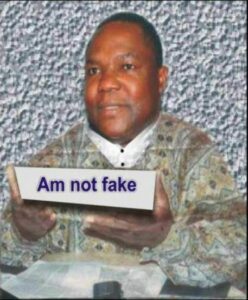
Delivery of fraudulent imagery: presentation attacks and injection attacks
There are two classes of attacks on biometric systems that aim to spoof biometrics by defeating liveness detection: presentation attacks and injection attacks.
Presentation attacks involve “presenting” non-live imagery to the camera during the capture process and representing it as live using physical media, such as paper photos and digital screens.
Injection attacks involve hardware or software hacks that enable a fraudster to “inject” digital imagery in such a way that bypasses a proper capture process with the primary camera.
Consider an analogous attack where a satellite transmission of a live speech by a political leader is intercepted and replaced with a digital deepfake video of them saying something else. The audience believes they are watching a live speech from their leader, when in fact they are watching a recorded speech from a deepfake saying something different. Injection attack detection helps mitigate this threat in the realm of biometric security.
Figure: a diagram of attack content, sites, and methods
The injection of fake imagery can take place at any of several attack sites within a device using either hardware or software hacks. Examples of injection attacks are:
Hardware attacks
|
Software attacks
|
Network attacks
|
Mitigating the risk: detecting attacks
Unlike the physical media used for presentation attacks, such as paper photos and screens, deepfakes and related digitally rendered content pose a different kind of threat. While deepfakes can be used in screen replay presentation attacks, these can be mitigated with PAD. But they can also be used in injection attacks that, as described above, bypass the camera and therefore warrant different detection methods.
With the evolution of deepfakes, countermeasures to combat it have advanced as well. There are numerous methods depending on the data available for analysis:
- Multi-modal analysis. AI analyzes lip movement and mouth shapes to detect discrepancies.
- Watermarking and digital signature techniques. These methods require calculating a hash or using a technique that embeds a hidden, digital signal or code within a video that can be used to identify the video’s origin.
- Video analysis. This includes a wide spectrum of different approaches based on machine learning and deep neural networks, including motion analysis, frame-by-frame analysis, and image artifact detectors.
- Digital injection attack detection. These methods are based on detecting the bypass of the normal capture process from a proper camera as described above. They may collect additional information from the operating system, browser, and whatever software and hardware is used for video capturing. A wide range of information is collected and compared with that known to be a proper capture and known to be an injection attack.
Summing up: a comprehensive approach to liveness includes detection of presentation and injection attacks
Ultimately, the importance of protecting biometric security from deepfakes and injection attacks cannot be overstated, given the rapid advancement and proliferation of deepfake rendering software. Deepfakes have the potential to seriously compromise biometric authentication, and it is crucial that organizations take steps to protect against them. By using presentation attack detection in concert with injection attack detection, organizations can better ensure the integrity of their systems.
Source: www.antispoofing.org